Overview
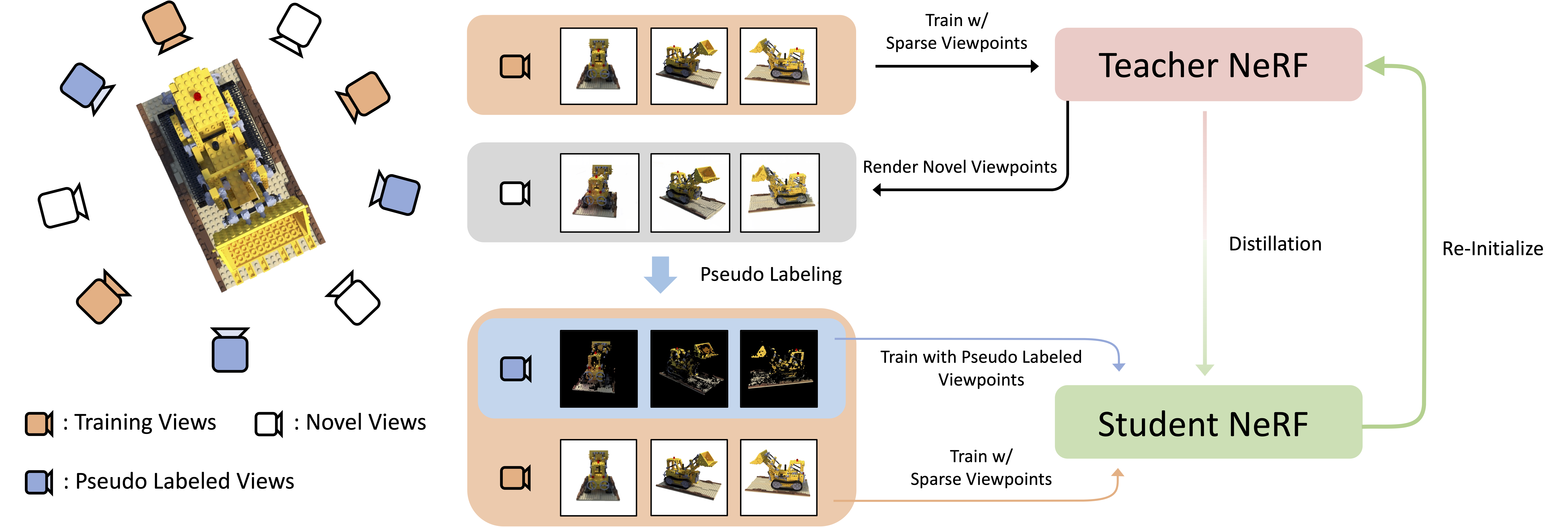
Abstract
Recently, neural radiance field (NeRF) has shown remarkable performance in novel view synthesis and 3D reconstruction. However, it still requires abundant high-quality images, limiting its applicability in real-world scenarios. To overcome this limitation, recent works have focused on training NeRF only with sparse viewpoints by giving additional regularizations, often called few-shot NeRF. We observe that due to the under-constrained nature of the task, solely using additional regularization is not enough to prevent the model from overfitting to sparse view- points. In this paper, we propose a novel framework, dubbed Self-Evolving Neural Radiance Fields (SE-NeRF), that applies a self-training framework to NeRF to address these problems. We formulate few-shot NeRF into a teacher-student frame- work to guide the network to learn a more robust representation of the scene by training the student with additional pseudo labels generated from the teacher. By distilling ray-level pseudo labels using distinct distillation schemes for reliable and unreliable rays obtained with our novel reliability estimation method, we enable NeRF to learn a more accurate and robust geometry of the 3D scene. We show and evaluate that applying our self-training framework to existing models improves the quality of the rendered images and achieves state-of-the-art performance in multiple settings.
Qualitative Results
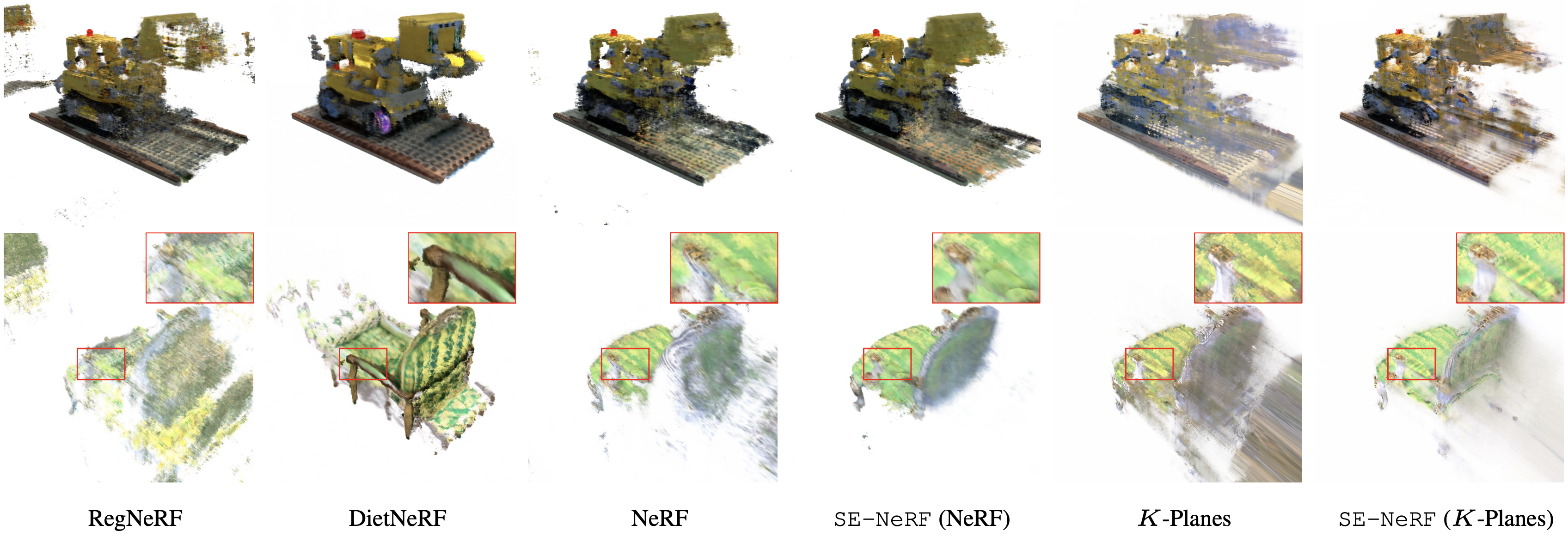
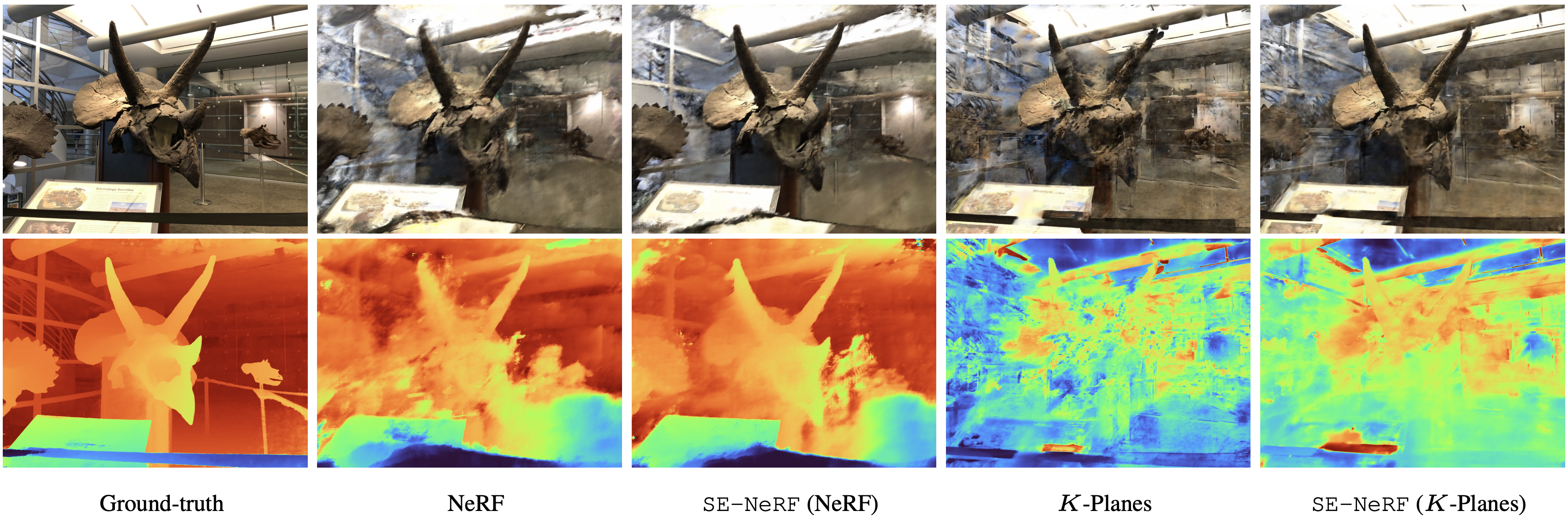
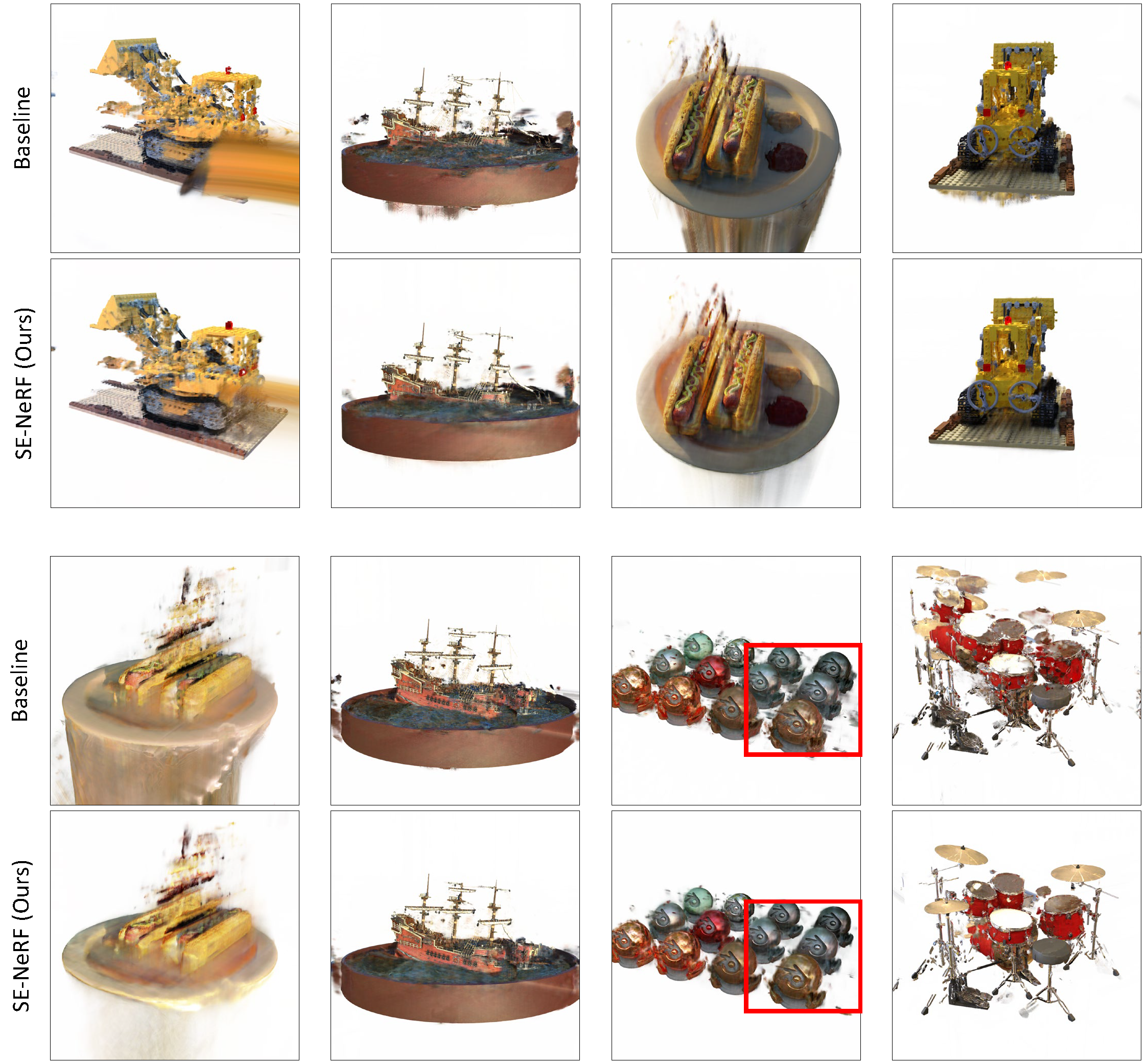
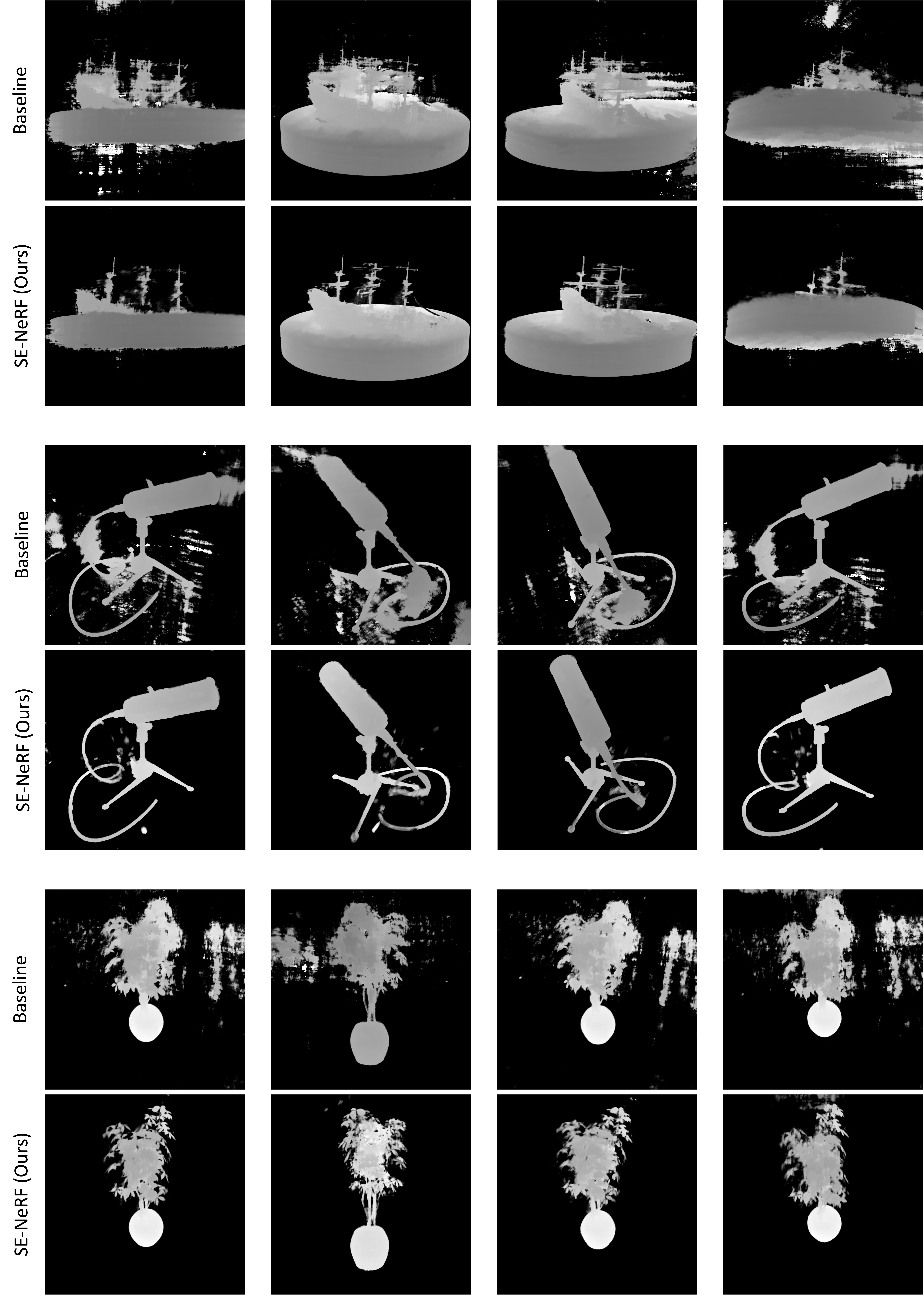
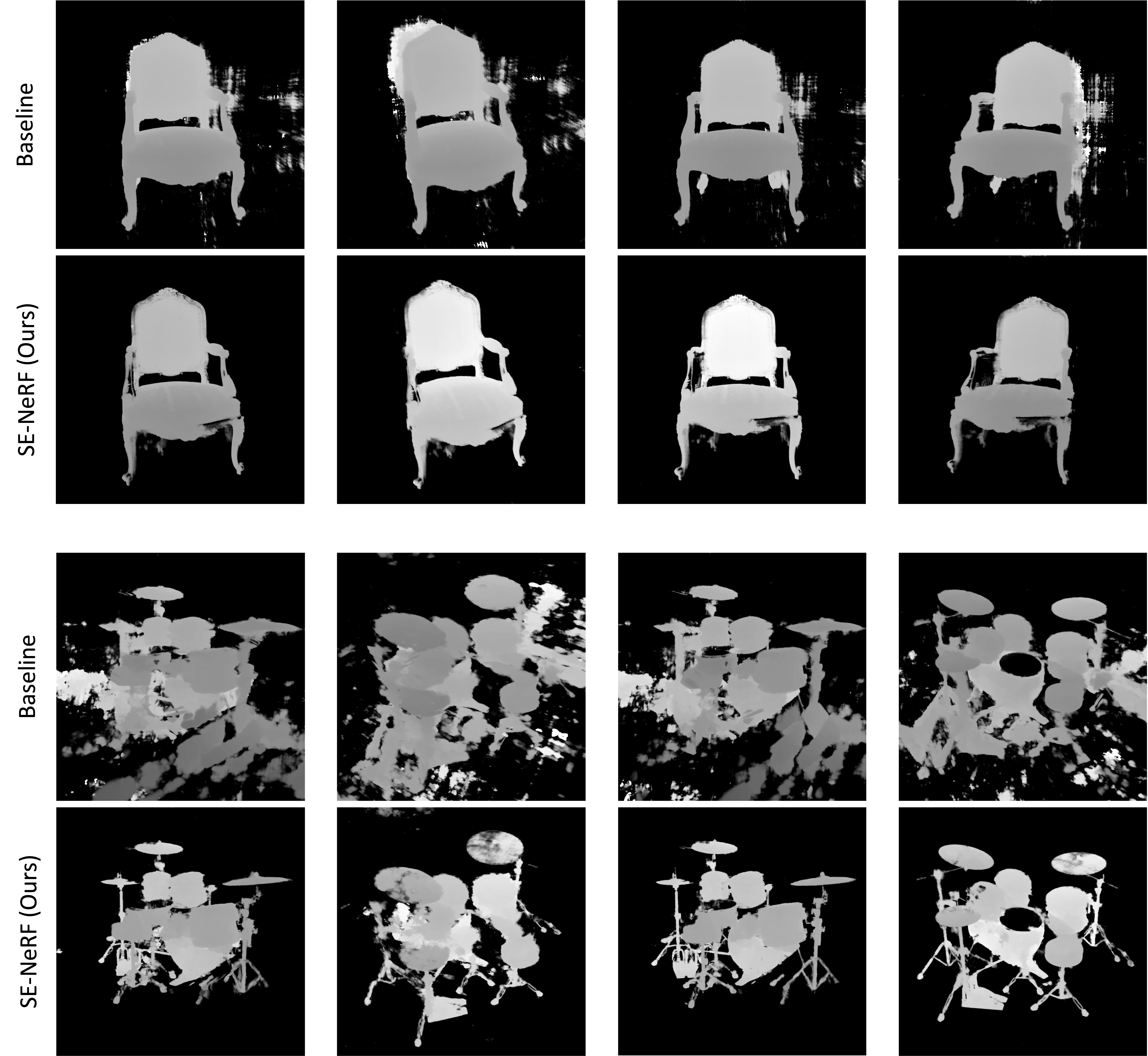
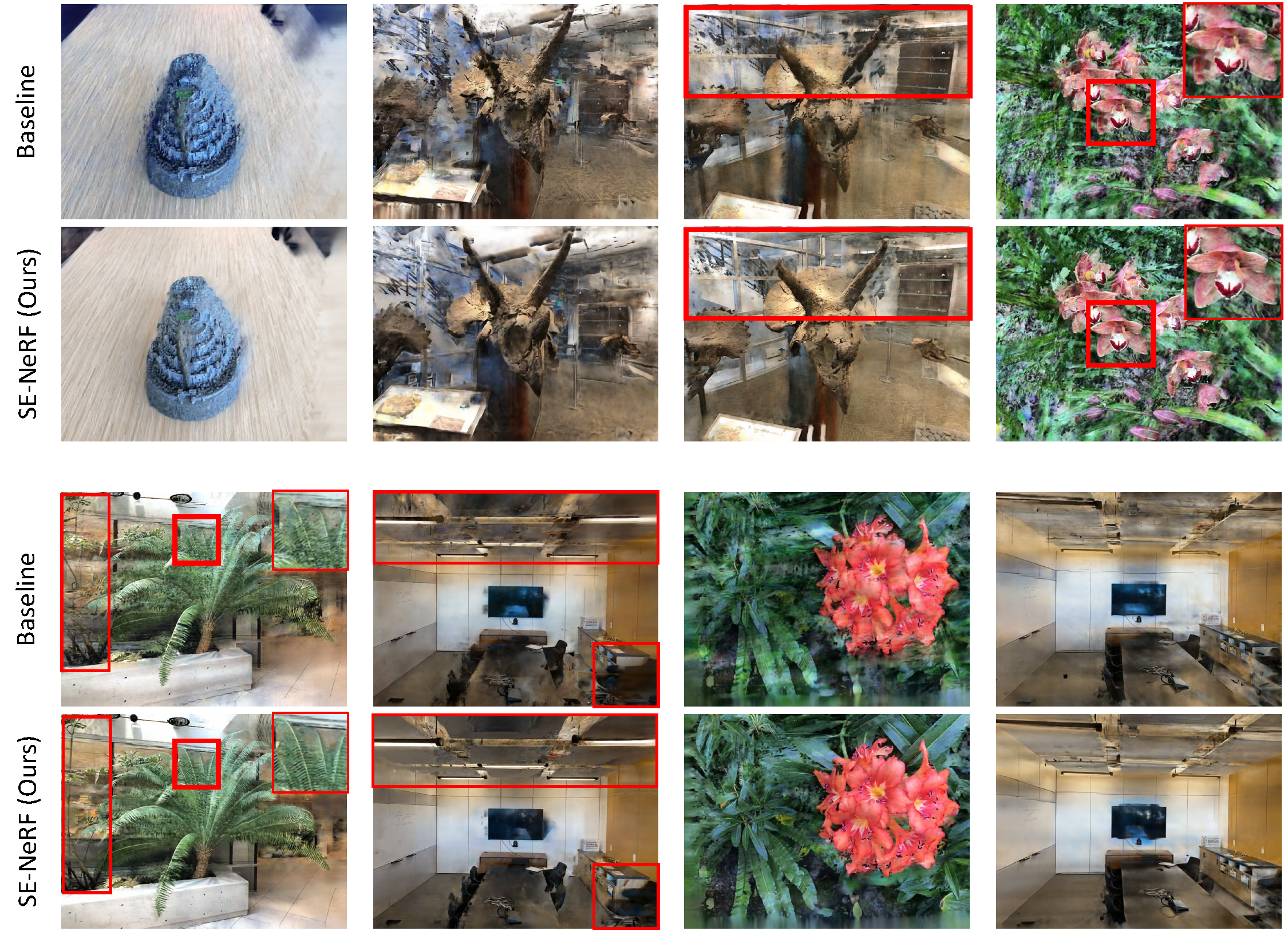
Quantitative Results
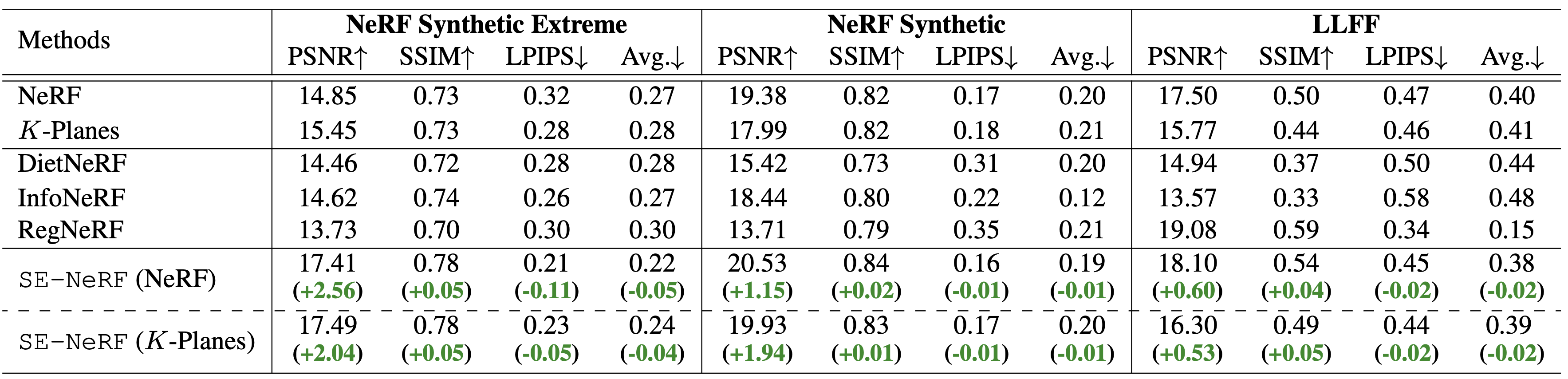
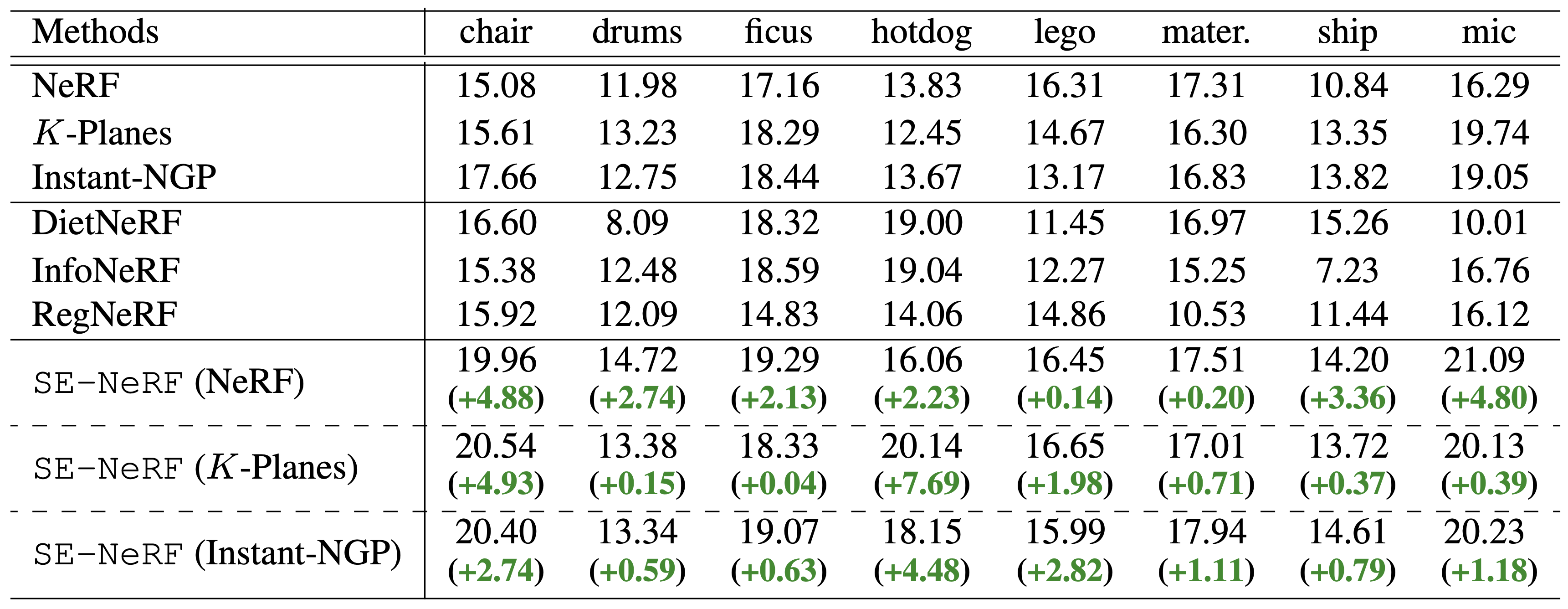
Motivation of Self-Supervised Training
Baseline: Trained with 3 images with the baseline model.
GT Reliability Mask: Trained with 3 images with self-supervised training applied with ground-truth reliability mask.
Ours(SE-NeRF): Trained with 3 images with our proposed self-supervised framework with no ground-truth information.
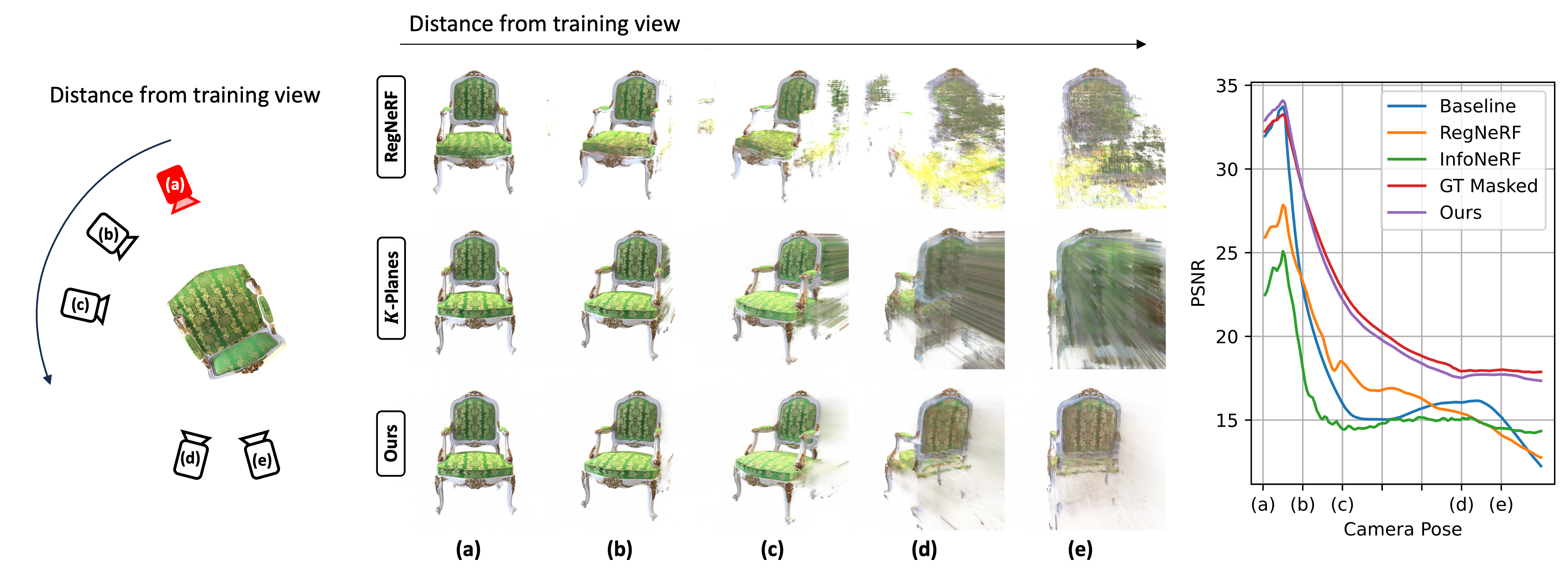
Citation
Acknowledgements
The website template was borrowed from Michaël Gharbi.